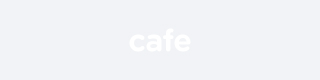
데이터 과학자를 위한 윤리/행동강령 (Code of Conduct) (nthought)
"윤리강령/행동강령이 필요하다는 글은 다수 발견됐지만 그런 강령을 문서로 잘 정리한 것은 발견하기 어려웠는데, 옥스퍼드대의 Dr. Peter Grindrod와 그라나다대의 Dr. Juan Bernabe Moreno라는 분이 2018년 3월에 정리해둔 강령이 있어서 (발)번역했습니다."
[ Oxford-Munich Code of Conduct for Professional Data Scientists ]
* Lawfulness (합법성)
1a. Lawful knowledge and adherence (법이해와 준법)
1b. Privacy and confidentiality
1c. Equality legislation (평등권)
* Competence (능력)
2a. Duty of Competence Development (능력 개발 의무)
2b. Competence communication transparency (능력 소통의 투명성)
* Dealing with Data (데이터 처리)
3a. Activity documentation: the personal lab book (활동 문서화: 개인 랩북)
3b. The Modeling Project Issues Log-Book (모델링 프로젝트의 이슈 로그북)
3b(i). Accuracy importance depending on the nature of the problem (문제의 본성에 따른 정확도 중요도)
3b(ii). Protocol and documentation (프로토콜과 문서화)
3b(iii). Data adequacy evaluation (데이터 타당성 평가)
3b(iv). Artificial data handling (가공 데이터 처리)
3b(v). Responsible data selection (책임있는 데이터 선택)
3b(vi). Inherent data bias (내재된 데이터 편향)
3b(vii). Surrogate feature and bias (대용 피쳐와 바이어스)
3c. Original data preservation (원본 데이터 보존)
3d. Collection vs use of data (수집과 데이터 사용)
3e. De-Identification (비식별화)
3f. Probabilistic (inferred) information and GDPR (확률 정보와 일반 데이터 보호 규제)
* Algorithms and models (알고리즘과 모델)
4a. Exhaustive algorithms: Data dredging, Data fishing, Data snooping, p-hacking (포괄적 알고리즘: 데이터 드렛징, 데이터 피싱, 데이터 스누핑, p-해킹)
4b. Sampling bias (샘플링 바이어스)
4c. Survivorship bias (생존 바이어스)
4d. Discarding unfavorable data (불리한 데이터 폐기)
4e. Causality and correlation (인과성와 상관성)
4f. Crisp geolocation analysis and Gerrymandering (크리스피 지역 분석과 게리만더링)
4g. Big picture beyond accuracy metrics (정확도 지표 너머의 큰 그림)
4h. Data Science and Publication bias (데이터 과학과 출판 바이어스)
4i. Accuracy vs. Explainability trade-off (정확도와 설명가능성 트레이드오프)
4j. Mandatory documentation of accuracy and precision and fit-for-purposeness (정확, 정밀, 목적부합의 필수 문서화)
4k. Pre-trained models re-usability (기존 학습 모델의 재사용)
4l. AI Reproducibility (AI 재현성)
4m. Cold-Start Bias (콜드스타트 바이어스)
4n. Prejudices and attempt against fundamental rights (편견과 기본권의 도전)
* Transparency Objectivity and Truth (투명성, 객관성, 진실)
5a. Transparency as a duty (투명성 의무)
5b. Provable objective results (입증가능한 객관적 결과)
5c. Clear results communication (명확한 결과 커뮤니케이션)
5d. Transparency on quality of the results (결과 품질의 투명성)
5e. Expectations alignment (기대 정열)
5f. Communication about dependency on data (데이터 의존도에 관한 커뮤니케이션)
5g. Liability in case of failure of the Data Scientist’s model (데이터 과학자의 모델 실패에 따른 책무)
5h. Creation of a manipulative evidence (조작 증거의 생성)
* Working alone and with others (혼자 또는 같이 일하기)
6a. Collegiability (평등성).
6b. Duty to speak up (강력 변호 의무)
6c. Code Awareness and adherence (강령 이해와 견지)
6d. Team code acquaintance and deviating behaviors (팀의 이해와 일탈 행위)
* extra) Upcoming ethical challenges (다가오는 윤리적 도전들)
7a. Adversarial Learning Manipulation (적대적 학습 조작)
7b. Responsibility on inventions (창작에 대한 책임)
7c. Explainable AI a research field and a duty (설명가능한 AI 연구와 의무)
7d. Blockchain and personal data (블록체인과 개인 데이터)
* 원문 : http://www.code-of-ethics.org/code-of-conduct/
* 출처(상세 내용 기술) : https://bahnsville.tistory.com/1164
******************************************************
- 통계분석연구회
- 카페 : http://cafe.daum.net/statsas
- 통계분석연구회(Statistics Analysis Study) 그룹
: https://www.facebook.com/groups/statsas
- STATWITH : http://statwith.tistory.com/
- RSS : https://statwith.tistory.com/rss
출처: https://statwith.tistory.com/entry/데이터-과학자를-위한-윤리행동강령-Code-of-Confuct-nthought [STATWITH]