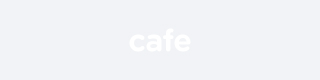
- nature
- scientific reports
- articles
- article
The vaginal microbiome and the risk of preterm birth: a systematic review and network meta-analysis
- Article
- Open access
- Published: 13 May 2022
The vaginal microbiome and the risk of preterm birth: a systematic review and network meta-analysis
- Unnur Gudnadottir,
- Justine W. Debelius,
- Juan Du,
- Luisa W. Hugerth,
- Hanna Danielsson,
- Ina Schuppe-Koistinen,
- Emma Fransson &
- Nele Brusselaers
Scientific Reports volume 12, Article number: 7926 (2022) Cite this article
7936 Accesses
35 Citations
35 Altmetric
Abstract
Preterm birth is a major cause of neonatal morbidity and mortality worldwide. Increasing evidence links the vaginal microbiome to the risk of spontaneous preterm labour that leads to preterm birth. The aim of this systematic review and network meta-analysis was to investigate the association between the vaginal microbiome, defined as community state types (CSTs, i.e. dominance of specific lactobacilli spp, or not (low-lactobacilli)), and the risk of preterm birth. Systematic review using PubMed, Web of Science, Embase and Cochrane library was performed. Longitudinal studies using culture-independent methods categorizing the vaginal microbiome in at least three different CSTs to assess the risk of preterm birth were included. A (network) meta-analysis was conducted, presenting pooled odds ratios (OR) and 95% confidence intervals (CI); and weighted proportions and 95% CI. All 17 studies were published between 2014 and 2021 and included 38–539 pregnancies and 8–107 preterm births. Women presenting with “low-lactobacilli” vaginal microbiome were at increased risk (OR 1.69, 95% CI 1.15–2.49) for delivering preterm compared to Lactobacillus crispatus dominant women. Our network meta-analysis supports the microbiome being predictive of preterm birth, where low abundance of lactobacilli is associated with the highest risk, and L. crispatus dominance the lowest.
조산은
전 세계적으로 신생아 이환율과 사망률의 주요 원인입니다.
조산으로 이어지는 자연 조기 진통의 위험과
질내 미생물군집이 연관되어 있다는 증거가 점점 더 많아지고 있습니다.
이 체계적 문헌고찰 및 네트워크 메타분석의 목적은
특정 유산균의 우세 여부(저유산균)와 같은
지역사회 상태 유형(CST)으로 정의되는
질내 미생물군과 조산의 위험 사이의 연관성을 조사하는 것이었습니다.
PubMed, 웹 오브 사이언스, 엠베이스 및 코크란 라이브러리를 사용한 체계적 문헌고찰을 수행했습니다. 조산 위험을 평가하기 위해 최소 세 가지 다른 CST에서 질내 미생물을 분류하는 배양 독립적 방법을 사용한 종단 연구가 포함되었습니다. 풀링된 오즈비(OR)와 95% 신뢰구간(CI), 가중치 비율과 95% CI를 제시하는 (네트워크) 메타분석이 수행되었습니다.
17개 연구는 모두 2014년부터 2021년 사이에 발표되었으며
38-539건의 임신과 8-107건의 조산이 포함되었습니다.
질 내 마이크로바이옴이 “저락토바실러스” 우세한 여성은 락토바실러스 크리스파투스 우세한 여성에 비해 조산 위험이 높았습니다(OR 1.69, 95% CI 1.15-2.49). 저희의 네트워크 메타 분석은 조산을 예측하는 마이크로바이옴을 뒷받침하며, 락토바실러스의 낮은 풍부도가 가장 높은 위험과 관련이 있고 L. 크리스파투스 우세도가 가장 낮은 것으로 나타났습니다.
Similar content being viewed by others
Article Open access20 December 2021
HIV-associated vaginal microbiome and inflammation predict spontaneous preterm birth in Zambia
Article Open access20 May 2022
Microbial diversity in the vaginal microbiota and its link to pregnancy outcomes
Article Open access04 June 2023
Introduction
Preterm birth (< 37 completed gestation weeks), which accounts for over 10% of births worldwide, is a major cause of neonatal mortality and morbidity1. Many factors can trigger premature labour onset, including preterm premature rupture of membranes (PPROM), infections (e.g. Trichomonas vaginalis and Chlamydia trachomatis2) and microbial invasion of the amniotic cavity3,4. The vaginal microbiome is thought to protect from such infections, with low diversity microbiome dominated by Lactobacillus species considered “healthy”. In contrast, a diverse microbiome with low abundance of lactobacilli and high amounts of anaerobic bacteria can cause dysbiosis, overlapping with the clinical bacterial vaginosis (BV) diagnosis5,6,7. BV is often asymptomatic, yet has been associated with higher risks of genital infections and complications, including human papillomavirus (HPV) infections8,9 and pelvic inflammatory disease10. It has also been proposed that different Lactobacillus species may present different risk profiles for various adverse events5,8. Since vaginal dysbiosis affects millions of women, it is important to understand the role of the vaginal microbiome in preterm birth5,11. Currently there are few studies available that assess the relationship between the vaginal microbiome and preterm birth, with conflicting findings on whether the vaginal microbiome can influence the risk of preterm birth2,12.
Although meta-analyses are a great tool to pool the results from different studies, common challenges are clinical and methodological heterogeneity. Meta-analysing microbiome studies is particularly difficult because of diverse study designs, limited power and a large variety in sampling and processing techniques, including different hypervariable regions targeted13,14. These challenges were described in a systematic review based on culture-independent methods to assess the vaginal microbiome and preterm birth, which included nine studies15. One systematic review included an individual-patient meta-analysis, yet only five cohorts had sequencing data publicly available16.
Our group recently introduced a novel method into the microbiome meta-analysis field to assess the relationship between the vaginal microbiome and the risk of HPV infections8. This network meta-analysis approach is based on aggregated data; and can be used to compare different microbiome “categories” in the same statistical model, based on direct and indirect evidence. Although categorizing the vaginal microbiome has its challenges, community state types (CSTs)6 are commonly used and easy to interpret.
We used this network-meta-analysis method to assess the association between the vaginal microbiome (as CSTs) and the risk of preterm birth, based on a comprehensive systematic review.
Methods
Study selection and criteria
Only longitudinal studies were considered, in which the vaginal microbiome was assessed clearly before the onset of labour, including premature rupture of membranes and other labour-associated complications; and in which all participants were followed up until delivery. Studies exclusively including high risk pregnancies were excluded to minimize the effects of risk factors apart from the microbiome (e.g., only women with prior preterm birth, cervical weakness). Original studies were eligible if they reported the risk of preterm birth in at least three CSTs or vaginal microbiome compositions6, with sufficient data to report the risk per individual and not per number of samples if multiple samples were collected per woman. The earliest pregnancy samples were used for the analysis if feasible. To enable the identification of species without the need for culturing, 16S analysis of samples was preferred. Since 16S sequencing techniques have only been available recently, only studies published since 2010 were included. As this study is based on aggregated data, we used the categorization of preterm and term delivery as reported in each paper, yet if possible, the categorization of the World Health Organization was used, defining preterm birth as birth before 37 completed weeks of gestation1.
We excluded intervention studies, cross-sectional studies with sampling after onset of labour, studies only investigating specific pathogens or only using culture-dependent or microscopic diagnostic methods. Reviews, editorial letters, case reports, conference abstracts, books, book chapters and commentaries were also excluded. We did not use language restrictions, to minimize the risk of language bias. No restrictions were used regarding the age of the included individuals or the study setting. If two or more studies presented the same cohort or overlapping cohorts the most recent study was included or both studies were considered as one study.
All results were reported according to the Preferred Reporting Items for Systematic Reviews and meta-analysis (PRISMA) extension for network meta-analysis17.
Information sources and search strategy
The search was conducted in PubMed, Web of Science, Embase and Cochrane Library and was last updated May 2021 (see search strings in Supplementary Table S1). The results were uploaded to EndNote X9 for the literature selection. The databases Prospero and Cochrane database of systematic reviews were searched to see if there were any ongoing studies on the subject.
The literature selection was conducted by two authors (UG & NB), by first removing all clearly irrelevant articles, followed by abstract and finally full text screening based on the eligibility criteria mentioned below.
Data extraction and assessment of risk of bias
We collected the following data (if available): study characteristics (country, setting, study design and period), study population (age range, race/ethnicity, recruitment, and specific inclusion criteria), information on exposure (i.e. factors that may affect the recorded vaginal microbiome composition: gestation week of sampling, CST, method of analysis and diversity measurements), and outcome characteristics (pregnancy week of birth, spontaneous or induced birth).
The quality of included studies was assessed by a customized checklist by two authors (UG & NB) (see Supplementary Table S2).
Data synthesis
Data used for the meta-analysis was extracted in double (UG, NB) to ensure quality, and meta-analyses were only conducted if at least three studies reported the required data.
We grouped the CSTs into five categories based on the dominating species: L. crispatus, L. gasseri, L. iners, “low-lactobacilli” and L. jensenii. “Low-lactobacilli” was defined as an increased diversity of anaerobic or a mixture of aerobe and facultative anaerobe bacteria (such as Gardnerella and Prevotella) based on the cut-offs and categorization used in the individual studies. CSTs which could not be transformed into these groups were omitted from the analysis. If possible, subgroup analyses were conducted based on study design, categorization of preterm birth (gestational week, spontaneous or not) and geographic region. These subgroups were chosen since spontaneous preterm birth could have different causes than induced preterm birth, and since the vaginal microbiome can differ depending on ethnicity/race6.
All analyses were conducted with Stata (MP 14, Stata Corporation), using the metaprop_one18 and network packages. The cumulative proportions of “low-lactobacilli” in each study were pooled and weighted using random effects models (to incorporate within-studies and between-studies variation)19, including the Freeman-Tukey double arcsine transformation to compute the weighted pooled estimate and to perform the back-transformation on the pooled estimate18.
To enable direct and indirect comparisons between all CSTs, we used a fixed network meta-analysis approach as described earlier8,20. This meta-analysis approach enables comparing different groups (CSTs) in the same statistical model in contrast to the classic pairwise meta-analysis only comparing two groups head-to-head.
A network map or network geometry20 was constructed to visualize all network relationships and available data on direct and indirect evidence available for the different CSTs, using crude data. The connection lines between the different dots indicate that direct information is available in at least one study, with thicker lines indicating that more studies report on this association. The larger the dots, the more studies present data on this specific CST. To assess if the results obtained by direct comparisons correspond to those obtained by indirect comparisons, the consistency of the models was measured. Large p-values (> 0.05) of the overall test and of the individual loop consistency tests imply that the consistency assumption can be accepted, and that this model can be used to give reliable assessments of the associations based on the available data. Forest plots were used to visualize and summarize the available evidence, presenting odds ratios (OR) and 95% confidence intervals (CI). In addition, the different CSTs were ranked depending on increasing risk of the outcomes, presented as relative probability which CST provides the “best outcome”, second best outcome, etc. These probability rankings should be interpreted with caution in observational settings with unbalanced groups. The number of included studies was too low for constructing funnel plots (to assess bias by small study effects).
In addition, average richness and diversity indices of each paper using either Chao1, Evenness (Simpson or Pielou) or Shannon index were pooled if sufficient data were available (at least 3 studies with means and standard deviations for both term and preterm pregnancies).
Results
Study selection
Out of 4321 unique articles, 17 cohort studies were included, all published in English between 2014 and 2021 (Fig. 1). None of the 79 retrieved studies in other languages were relevant. The number of pregnancies per study ranged between 38 and 539, with 8 and 107 preterm births.
Figure 1
PRISMA flowchart of selection of articles included in the network meta-analysis.
The most common exclusion criteria of otherwise eligible studies were the lack of CST grouping of results (Supplementary Table S3). In the final selection of studies, seven were excluded because a lack of CST grouping11,21,22,23,24,25,26, all women receiving cervical cerclage27, sampling after signs of labor28, no information regarding preterm birth for current pregnancy29, only the use of polymerase chain reaction (PCR) instead of sequencing30 or multiple CSTs assigned to each woman because of multiple sampling points31.
Study characteristics and quality
Out of the 17 eligible studies, seven originated from North-America4,5,32,33,34,35,36, three from Europe37,38,39, two from South-America40,41, three from Asia42,43,44 and two from Africa45,46. Microbiome samples were taken before the third trimester in all studies. Five studies specified that women at high-risk of preterm birth were not excluded from the cohort37,38,39,43,45, while others did not specify the risk profiles. Out of the five studies that included high risk women, one study included 29 HIV positive women45, one included women diagnosed with preterm prelabour rupture of membranes (PPROM)43 and three included unspecified high-risk women37,38,39.
Preterm birth was defined as birth before 37 completed weeks of gestation for all studies except one, where it was defined as before 34 weeks of gestation4. In twelve studies, a healthcare professional took the samples4,5,32,37,38,39,41,42,43,44,45,46, while the other five had self-sampling33,34,35,36,40. Furthermore, all studies except for two reported that the onset of preterm birth was spontaneous (Supplementary Table S4)45,46.
All studies used 16S analysis of the microbiome samples, except one which used shotgun sequencing37. Among the 16S studies, four hypervariable regions of the 16S molecule were targeted, with most studies targeting either the V1-3 or V3-4 hypervariable regions (Supplementary Table S4). The studies reported up to 13 different CSTs, which were re-categorized as mentioned above. Shannon diversity index was reported in 15 out of the 17 studies4,5,32,33,34,35,36,37,38,40,42,43,44,45,46, but it varied if mean or median value was used, if standard deviation was reported and if the value was reported as number or as figure, so pooling was not feasible. The other diversity measures were not reported frequently enough to pool the results.
Synthesis of results
Among women who delivered preterm, the pooled proportion with “low-lactobacilli” was 0.41 (95% CI 0.30–0.53) compared to 0.29 (95% CI 0.20–0.38) of women with term deliveries (Fig. 2).
Figure 2
Forest plots showing all 17 included studies and the pooled and weighted proportion of “low-lactobacilli” women who delivered (a) preterm and (b) at term.
The network map (Fig. 3) indicates that direct evidence was available for the association between all five CST categories (at least eight studies reported on each CST category). The test for inconsistency indicated overall consistency (p = 0.77), and so did all loop inconsistency tests (p > 0.05), indicating this method can be used to assess the associations between the different CSTs.
Figure 3
Network map of all 17 included studies by vaginal microbiome composition, showing how many studies reported which community state types (CSTs). Legend: Each dot represents a CST, with the number indicating how many studies reported it. The lines between the blue dots and their thickness represent the number of studies which reported the CSTs joined by the line.
The risk of preterm birth was higher among women presenting with “low-lactobacilli” compared to L. crispatus (OR 1.69, 95% CI 1.15–2.49) (Fig. 4). The risk of preterm birth was also high among women with L. jensenii compared to L. crispatus (OR 1.68, 95% CI 0.97–2.92), yet these results did not reach statistical significance.
Figure 4
Forest plots comparing community state types (CSTs) and their risk of preterm birth using (a) Lactobacillus crispatus and (b) “Low-lactobacilli” as reference group, where an odds ratio (OR) > 1.00 indicates association with preterm birth.
Ranking tests showed that the L. crispatus dominant group was most probable to be the “best” microbiome composition, and L. jensenii the most probably the “worst” group considering the association with preterm birth (figure not shown).
Subgroup analyses
Four different subgroup analyses were conducted: (1) Preterm birth defined < 37 weeks (excluding the one study only including early preterm birth)5,32,33,34,36,37,38,39,40,41,42,43,44,45,46, (2) Clear spontaneous preterm birth4,5,32,33,34,36,37,38,39,40,41,42,43,44, (3) Region North-America and Europe4,5,32,33,34,35,36,37,38,39 and (4) Region South-America, Asia and Africa40,41,42,43,44,45,46 (Table 1). These subgroup analyses showed consistent results with the overall analysis, although the analyses for preterm birth as < 37 weeks had insufficient power (Table 1).
Table 1 Subgroup analysis by definitions of preterm birth, geographical region and if all cases of preterm birth were clearly spontaneous.
Discussion
This network meta-analysis suggests that women with a “low-lactobacilli” vaginal microbiome composition were at higher risk of preterm birth (spontaneous and overall) compared to women with L. crispatus dominant microbiome compositions.
Our systematic review and network meta-analysis is the first of its kind, since only one meta-analysis had previously been done on this subject, which used individual level sequencing data16. We chose CSTs over individual sequencing data because there can be a lack of open access to the data leading to selection bias of studies, and updating the recently published individual patient data meta-analysis would not have contributed any new information to the field. Furthermore, CSTs are more complimentary to the current knowledge, and although not ideal, are good for clinical uses and for identifying targets for future developments. Therefore, we see both meta-analysis approaches as complementary. Although we also had to exclude six otherwise eligible studies because CSTs were not reported, we were able to include 17 studies, compared to the six studies of the previous meta-analysis16 (only one study in common4). Authors of the excluded papers were contacted for data but never replied. As mentioned above, heterogeneity of methods may propose problems and decrease the number of studies which can be included in individual patient data meta-analyses. Nonetheless, by using CST categorization we were able to compare five different groups (CSTs) to each other in this network approach, instead of just using two groups as is common in classic meta-analyses. The use of CSTs was first described in a small cohort study from 20106 and has been widely used despite its challenges and limitations47,48, but it is currently the best option in the field to categorize vaginal microbiome compositions. Many of the included studies used an adapted form of the original CSTs, using a range from 3 to 13 groups. Most common were subgroups of the diverse non-lactobacilli dominant group, but there was not enough uniformity between those in the studies to use subgrouping for this analysis. Despite these factors, our inconsistency tests gave robust results showing that the method is stable enough to use the results.
When comparing microbiome studies, there are always many factors that can influence the results, such as the sample collection, extraction methods and hypervariable region used when sequencing13. The motivation for hypervariable region selection varies, with emphasis placed on universality or specificity. The V4 hypervariable region is more conserved among Lactobacillus species, making species-level assignments more uncertain. In contrast, the long amplicons generated by the V1-V3 hypervariable region can be challenging for short-read technologies49.
Different studies may also have different cut-off levels for what defines a “dominant” group, and that cut-off is seldom specified. Furthermore, in the included studies the social or physical underlying risk of preterm birth of the women was not always well-defined or incorporated in the analyses.
Our results are consistent with the previously conducted meta-analysis16, which showed that women that delivered preterm had higher diversity in their vaginal microbiome, which is associated with the “low-lactobacilli” CST. The reviews on this subject also agree that even though current studies are not all consistent, it seems that overall L. crispatus is protective and a “low-lactobacilli” microbiome might increase the risk of spontaneous preterm birth2,12,15. Furthermore, as many of the included studies were published after the systematic-review of the topic15, better methods and technologies might account for why more studies are now finding association between the microbiome and PTB. Yet, several of the included studies were still hampered by low sample sizes, methodological heterogeneity and selection bias (as seen by the unrepresentatively high proportions of preterm birth cases in some studies).
The “low-lactobacilli” group includes bacterial species such as Gardnerella and Prevotella, both of which are known to promote proinflammatory cytokines and are commonly found in the vaginal microbiome just before PPROM12. It is therefore not surprising that they may have an impact on the maternal immune response and play a part in inducing preterm birth.
These results are important for the possible prediction and prevention of preterm birth which remains an important problem today. Yet, further longitudinal studies are needed to incorporate potential natural changes in the vaginal microbiome during pregnancy4, and to better understand the pathophysiological mechanisms underlying these apparent different risk profiles.
Conclusion
To conclude, the diversity of the vaginal microbiome seems to play a part in the risk of preterm birth, where women with low abundance of lactobacilli were at greater risk of delivering preterm compared to women with L. crispatus dominant microbiome.
Data availability
All data is available from the included articles and in the Supplement.
References
(WHO) WHO. Preterm Birth. https://www.who.int/news-room/fact-sheets/detail/preterm-birth.
Bayar, E., Bennett, P. R., Chan, D., Sykes, L. & MacIntyre, D. A. The pregnancy microbiome and preterm birth. Semin. Immunopathol. 42(4), 487–499 (2020).
Baldwin, E. A. et al. Persistent microbial dysbiosis in preterm premature rupture of membranes from onset until delivery. PeerJ 3, e1398 (2015).
Romero, R. et al. The vaginal microbiota of pregnant women who subsequently have spontaneous preterm labor and delivery and those with a normal delivery at term. Microbiome 2, 1–15 (2014).
Fettweis, J. M. et al. The vaginal microbiome and preterm birth. Nat. Med. 25(6), 1012 (2019).
Ravel, J. et al. Vaginal microbiome of reproductive-age women. Proc. Natl. Acad. Sci. USA 15(108 Suppl 1), 4680–4687 (2011).
van de Wijgert, J. H. et al. The vaginal microbiota: What have we learned after a decade of molecular characterization?. PLoS ONE 9(8), e105998 (2014).
Norenhag, J. et al. The vaginal microbiota, human papillomavirus and cervical dysplasia: A systematic review and network meta-analysis. BJOG 127(2), 171–180 (2020).
Brusselaers, N., Shrestha, S., van de Wijgert, J. & Verstraelen, H. Vaginal dysbiosis and the risk of human papillomavirus and cervical cancer: Systematic review and meta-analysis. Am. J. Obstet. Gynecol. 221(1), 9–18 (2019).
Chen, X., Lu, Y., Chen, T. & Li, R. The female vaginal microbiome in health and bacterial vaginosis. Front. Cell. Infect. Microbiol. 11, 631972 (2021).
Hyman, R. W. et al. Diversity of the Vaginal Microbiome Correlates With Preterm Birth. Reprod Sci. 21(1), 32–40 (2014).
Tsonis, O. et al. Female genital tract microbiota affecting the risk of preterm birth: What do we know so far? A review. Eur. J. Obstet. Gynecol. Reprod. Biol. 245, 168–173 (2020).
Hugerth, L. W. & Andersson, A. F. Analysing microbial community composition through amplicon sequencing: From sampling to hypothesis testing. Front. Microbiol. 8, 1561 (2017).
Debelius, J. et al. Tiny microbes, enormous impacts: What matters in gut microbiome studies?. Genome Biol. 17(1), 217 (2016).
Peelen, M. et al. The influence of the vaginal microbiota on preterm birth: A systematic review and recommendations for a minimum dataset for future research. Placenta 79, 30–39 (2019).
Kosti, I., Lyalina, S., Pollard, K. S., Butte, A. J. & Sirota, M. Meta-analysis of vaginal microbiome data provides new insights into preterm birth. Front. Microbiol. 11, 476. https://doi.org/10.3389/fmicb.2020.00476 (2020).
Hutton, B. et al. The PRISMA extension statement for reporting of systematic reviews incorporating network meta-analyses of health care interventions: Checklist and explanations. Ann. Intern. Med. 162(11), 777–784 (2015).