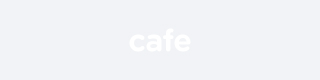
신동광. (2022). 채점자질의 적용이 영어 자동채점 모델의 성능에 미치는 영향. 교원교육, 38(6), 73-91.
연구목적: 본 연구는 채점자질(rating features)을 적용하는 자동채점 모델과 채점자질을 사용하지 않는 자동채점 모델 간의 성능을 비교하는 데 목적이 있다.
연구방법: 연구에 활용된 데이터는 kaggle 사이트에서 다운로드 받은 영어 원어민 10학년 수준의 학습자들 이 작성한 300개의 에세이 답안이며 이를 7:3의 비율로 학습용 데이터와 검증용 데이터로 구분하였다. 답안은 사전에 인간 채점자들이 이미 6개 채점영역별로 0~6점의 척도에 기반한 채점기준표를 활용하여 분석적 채점을 수행한 데이터였다. 데이터 분류 결과, 에세이 답안이 극히 희소한 점수대는 인공지능 학습이 사실상 불가능하여 제외하고 3~5점대 답안만 실험에 활용하였다. 먼저 채점자질을 활용할 수 있는 랜덤포레스트(RF) 모델을 활용하여 Coh-Metrix 분석에서 추출한 106개 언어자질값을 기계학습시켜 자동채점 결과를 산출하였다. 그 다음, 채점자질을 사용하지 않는 딥러닝 계열의 3개 모델인 순환신경망(RNN), 장단기기억(LSTM), 게이트순환유닛(GRU)에 기반한 자동채점 결과를 산출하였다. 이를 바탕으로 4개 자동채점 모델 및 인간 채점의 결과에 대해 정확도(accuracy)를 기준으로 일치 정도를 비교하였다. 끝으로 랜덤포레스트 모델의 경우 어떠한 채점자질이 채점 결과에 영향을 미치는 주요 요인인지를 추가 분석하였다.
연구결과: 딥러닝 계열인 RNN의 경우는 인간 채점 결과와의 일치도가 채점영역별로 .39~.69(평균=.58)로 나타났고 LSTM은 .59~.72(평균=.64), GRU는 .59~.72(평균=.64)로 나타났다. 반면 채점자질을 기계학습한 RF의 정확도 결과는 .60~.76(평균=.69)로 인간 채점 결과와 가장 높은 일치도를 보였다. RF 모델의 경우 ‘단어수,’ ‘문장수,’ ‘어휘밀도’ 등이 전체 채점영역에서 공통적으로 주요한 자질로 나타났으며 각 채점영역의 특성이 반영된 주요 채점자질들도 다수 확인되었다.
결론: 대규모의 데이터 확보가 어려운 상황에서는 RF 모델이 상대적으로 높은 자동채점 성능을 보였으며 자동채점 결과의 활용 면에서도 채점자질의 분석값에 기반한 교수⋅학습적 피드백 제공이 가능한 RF 방식이 타 채점 보델과 비교하여 상대적으로 더 유용할 것으로 판단된다.
주제어: 자동채점, 채점자질, 기계학습, 딥러닝, 랜덤포레스트
교원교육 38권 6호
Korean Journal of Teacher Education, Vol. 38, No. 6 pp. 73-91
https://doi.org/10.14333/KJTE.2022.38.6.04
pISSN 1225-2042
eISSN 2288-5706
Effects of Scoring Features on the Accuracy of the Automated Scoring Model of English
by Shin, Dong-Kwang
Abstract
Purpose: This study attempted to compare the performance of an automated scoring model based on scoring features and three other automated scoring models that do not use the scoring feature.
Methods: The data used in this study were 300 essays written by native English speakers in the tenth grade, which were divided into training and validating data at a ratio of 7:3. The RF model was used to predict the scores of the essays based on the analysis of 106 linguistic features extracted from Coh-etrix. The accuracy of this model was compared to that of three deep learning models―RNN, LSTM, and GRU―which do not use those scoring features. Lastly, in the case of the RF model, scoring features mainly affecting accuracy prediction were further analyzed.
Results: RNN which is a type of deep learning had an agreement with human scoring results ranging from .39 to .69 (mean=.58) in each rating domain, while LSTM showed an agreement between .59 and .72 (mean=.64), and GRU’s agreement was between .59 and .72 (mean=.64). However, when using the RF model based on the scoring features, its average accuracy was between .60 to .76 (mean=.69), which is the highest agreement among the four models. In the case of the RF model, ‘word count,’ ‘sentence count,’ and ‘vocabulary density’ were common characteristics across all scoring domains, but several scoring features reflecting the characteristics of each scoring domain were also found.
Conclusion: When large-scale data is not available, the RF model showed relatively high automated scoring performance, and the RF model that provides learners with pedagogical feedback based on scoring features would be more useful compared to the other three automated scoring models in terms of the utilization of the automated scoring result.
Key words: automated scoring, scoring feature, machine learning, deep learning, random forest